visualkeras: transformers example#
An example showing the visualkeras
function
used by a tf.keras.Model
or Module
or
TFPreTrainedModel
model.
# Authors: The scikit-plots developers
# SPDX-License-Identifier: BSD-3-Clause
Force garbage collection
import gc
gc.collect()
3
# pip install protobuf==5.29.4
import tensorflow as tf
# Clear any session to reset the state of TensorFlow/Keras
tf.keras.backend.clear_session()
from transformers import TFAutoModel
from scikitplot import visualkeras
# Load the Hugging Face transformer model
transformer_model = TFAutoModel.from_pretrained("microsoft/mpnet-base")
# Define a Keras-compatible wrapper for the Hugging Face model
def wrap_transformer_model(inputs):
input_ids, attention_mask = inputs
outputs = transformer_model(input_ids=input_ids, attention_mask=attention_mask)
return outputs.last_hidden_state # Return the last hidden state for visualization
Some weights of the PyTorch model were not used when initializing the TF 2.0 model TFMPNetModel: ['lm_head.dense.bias', 'lm_head.decoder.bias', 'lm_head.bias', 'lm_head.layer_norm.weight', 'lm_head.dense.weight', 'lm_head.layer_norm.bias', 'lm_head.decoder.weight']
- This IS expected if you are initializing TFMPNetModel from a PyTorch model trained on another task or with another architecture (e.g. initializing a TFBertForSequenceClassification model from a BertForPreTraining model).
- This IS NOT expected if you are initializing TFMPNetModel from a PyTorch model that you expect to be exactly identical (e.g. initializing a TFBertForSequenceClassification model from a BertForSequenceClassification model).
Some weights or buffers of the TF 2.0 model TFMPNetModel were not initialized from the PyTorch model and are newly initialized: ['mpnet.pooler.dense.weight', 'mpnet.pooler.dense.bias']
You should probably TRAIN this model on a down-stream task to be able to use it for predictions and inference.
# Define Keras model inputs
input_ids = tf.keras.Input(shape=(128,), dtype=tf.int32, name="input_ids")
attention_mask = tf.keras.Input(shape=(128,), dtype=tf.int32, name="attention_mask")
# Pass inputs through the transformer model using a Lambda layer
last_hidden_state = tf.keras.layers.Lambda(
wrap_transformer_model,
output_shape=(128, 768), # Explicitly specify the output shape
name="microsoft_mpnet-base",
)([input_ids, attention_mask])
# Reshape the output to fit into Conv2D (adding extra channel dimension) inside a Lambda layer
# def reshape_last_hidden_state(x):
# return tf.reshape(x, (-1, 1, 128, 768))
# reshaped_output = tf.keras.layers.Lambda(reshape_last_hidden_state)(last_hidden_state)
# Use Reshape layer to reshape the output to fit into Conv2D (adding extra channel dimension)
# Reshape to (batch_size, 128, 768, 1) for Conv2D input
reshaped_output = tf.keras.layers.Reshape((-1, 128, 768))(last_hidden_state)
# Add different layers to the model
x = tf.keras.layers.Conv2D(
512, (3, 3), activation="relu", padding="same", name="conv2d_1"
)(reshaped_output)
x = tf.keras.layers.BatchNormalization(name="batchnorm_1")(x)
x = tf.keras.layers.Dropout(0.3, name="dropout_1")(x)
x = tf.keras.layers.MaxPooling2D(pool_size=(2, 2), name="maxpool_1")(x)
x = tf.keras.layers.Conv2D(
256, (3, 3), activation="relu", padding="same", name="conv2d_2"
)(x)
x = tf.keras.layers.BatchNormalization(name="batchnorm_2")(x)
x = tf.keras.layers.Dropout(0.3, name="dropout_2")(x)
x = tf.keras.layers.MaxPooling2D(pool_size=(2, 2), name="maxpool_2")(x)
x = tf.keras.layers.Conv2D(
128, (3, 3), activation="relu", padding="same", name="conv2d_3"
)(x)
x = tf.keras.layers.BatchNormalization(name="batchnorm_3")(x)
x = tf.keras.layers.Dropout(0.4, name="dropout_3")(x)
x = tf.keras.layers.MaxPooling2D(pool_size=(2, 2), name="maxpool_3")(x)
# Add GlobalAveragePooling2D before the Dense layers
x = tf.keras.layers.GlobalAveragePooling2D(name="globalaveragepool")(x)
# Add Dense layers
x = tf.keras.layers.Dense(512, activation="relu", name="dense_1")(x)
x = tf.keras.layers.Dropout(0.5, name="dropout_4")(x)
x = tf.keras.layers.Dense(128, activation="relu", name="dense_2")(x)
# Add output layer (classification head)
dummy_output = tf.keras.layers.Dense(
2, activation="softmax", name="dummy_classification_head"
)(x)
# Wrap into a Keras model
wrapped_model = tf.keras.Model(inputs=[input_ids, attention_mask], outputs=dummy_output)
# https://github.com/keras-team/keras/blob/v3.3.3/keras/src/models/model.py#L217
# https://github.com/keras-team/keras/blob/master/keras/src/utils/summary_utils.py#L121
wrapped_model.summary(
line_length=None,
positions=None,
print_fn=None,
expand_nested=False,
show_trainable=True,
layer_range=None,
)
Model: "functional"
┏━━━━━━━━━━━━━━━━━━━┳━━━━━━━━━━━━━━━━━┳━━━━━━━━━━━┳━━━━━━━━━━━━━━━━┳━━━━━━━┓
┃ Layer (type) ┃ Output Shape ┃ Param # ┃ Connected to ┃ Trai… ┃
┡━━━━━━━━━━━━━━━━━━━╇━━━━━━━━━━━━━━━━━╇━━━━━━━━━━━╇━━━━━━━━━━━━━━━━╇━━━━━━━┩
│ input_ids │ (None, 128) │ 0 │ - │ - │
│ (InputLayer) │ │ │ │ │
├───────────────────┼─────────────────┼───────────┼────────────────┼───────┤
│ attention_mask │ (None, 128) │ 0 │ - │ - │
│ (InputLayer) │ │ │ │ │
├───────────────────┼─────────────────┼───────────┼────────────────┼───────┤
│ microsoft_mpnet-… │ (None, 128, │ 0 │ input_ids[0][… │ - │
│ (Lambda) │ 768) │ │ attention_mas… │ │
├───────────────────┼─────────────────┼───────────┼────────────────┼───────┤
│ reshape (Reshape) │ (None, 1, 128, │ 0 │ microsoft_mpn… │ - │
│ │ 768) │ │ │ │
├───────────────────┼─────────────────┼───────────┼────────────────┼───────┤
│ conv2d_1 (Conv2D) │ (None, 1, 128, │ 3,539,456 │ reshape[0][0] │ Y │
│ │ 512) │ │ │ │
├───────────────────┼─────────────────┼───────────┼────────────────┼───────┤
│ batchnorm_1 │ (None, 1, 128, │ 2,048 │ conv2d_1[0][0] │ Y │
│ (BatchNormalizat… │ 512) │ │ │ │
├───────────────────┼─────────────────┼───────────┼────────────────┼───────┤
│ dropout_1 │ (None, 1, 128, │ 0 │ batchnorm_1[0… │ - │
│ (Dropout) │ 512) │ │ │ │
├───────────────────┼─────────────────┼───────────┼────────────────┼───────┤
│ maxpool_1 │ (None, 0, 64, │ 0 │ dropout_1[0][… │ - │
│ (MaxPooling2D) │ 512) │ │ │ │
├───────────────────┼─────────────────┼───────────┼────────────────┼───────┤
│ conv2d_2 (Conv2D) │ (None, 0, 64, │ 1,179,904 │ maxpool_1[0][… │ Y │
│ │ 256) │ │ │ │
├───────────────────┼─────────────────┼───────────┼────────────────┼───────┤
│ batchnorm_2 │ (None, 0, 64, │ 1,024 │ conv2d_2[0][0] │ Y │
│ (BatchNormalizat… │ 256) │ │ │ │
├───────────────────┼─────────────────┼───────────┼────────────────┼───────┤
│ dropout_2 │ (None, 0, 64, │ 0 │ batchnorm_2[0… │ - │
│ (Dropout) │ 256) │ │ │ │
├───────────────────┼─────────────────┼───────────┼────────────────┼───────┤
│ maxpool_2 │ (None, 0, 32, │ 0 │ dropout_2[0][… │ - │
│ (MaxPooling2D) │ 256) │ │ │ │
├───────────────────┼─────────────────┼───────────┼────────────────┼───────┤
│ conv2d_3 (Conv2D) │ (None, 0, 32, │ 295,040 │ maxpool_2[0][… │ Y │
│ │ 128) │ │ │ │
├───────────────────┼─────────────────┼───────────┼────────────────┼───────┤
│ batchnorm_3 │ (None, 0, 32, │ 512 │ conv2d_3[0][0] │ Y │
│ (BatchNormalizat… │ 128) │ │ │ │
├───────────────────┼─────────────────┼───────────┼────────────────┼───────┤
│ dropout_3 │ (None, 0, 32, │ 0 │ batchnorm_3[0… │ - │
│ (Dropout) │ 128) │ │ │ │
├───────────────────┼─────────────────┼───────────┼────────────────┼───────┤
│ maxpool_3 │ (None, 0, 16, │ 0 │ dropout_3[0][… │ - │
│ (MaxPooling2D) │ 128) │ │ │ │
├───────────────────┼─────────────────┼───────────┼────────────────┼───────┤
│ globalaveragepool │ (None, 128) │ 0 │ maxpool_3[0][… │ - │
│ (GlobalAveragePo… │ │ │ │ │
├───────────────────┼─────────────────┼───────────┼────────────────┼───────┤
│ dense_1 (Dense) │ (None, 512) │ 66,048 │ globalaverage… │ Y │
├───────────────────┼─────────────────┼───────────┼────────────────┼───────┤
│ dropout_4 │ (None, 512) │ 0 │ dense_1[0][0] │ - │
│ (Dropout) │ │ │ │ │
├───────────────────┼─────────────────┼───────────┼────────────────┼───────┤
│ dense_2 (Dense) │ (None, 128) │ 65,664 │ dropout_4[0][… │ Y │
├───────────────────┼─────────────────┼───────────┼────────────────┼───────┤
│ dummy_classifica… │ (None, 2) │ 258 │ dense_2[0][0] │ Y │
│ (Dense) │ │ │ │ │
└───────────────────┴─────────────────┴───────────┴────────────────┴───────┘
Total params: 5,149,954 (19.65 MB)
Trainable params: 5,148,162 (19.64 MB)
Non-trainable params: 1,792 (7.00 KB)
# Visualize the wrapped model
img_nlp_mpnet_with_tf_layers = visualkeras.layered_view(
wrapped_model,
legend=True,
show_dimension=True,
min_z=1,
min_xy=1,
max_z=4096,
max_xy=4096,
scale_z=1,
scale_xy=1,
font={"font_size": 99},
text_callable="default",
# to_file="result_images/nlp_mpnet_with_tf_layers.png",
save_fig=True,
save_fig_filename="nlp_mpnet_with_tf_layers.png",
overwrite=False,
add_timestamp=True,
verbose=True,
)
img_nlp_mpnet_with_tf_layers
<PIL.Image.Image image mode=RGBA size=6879x3530 at 0x7FB29473FDD0>
Total running time of the script: (0 minutes 5.721 seconds)
Related examples
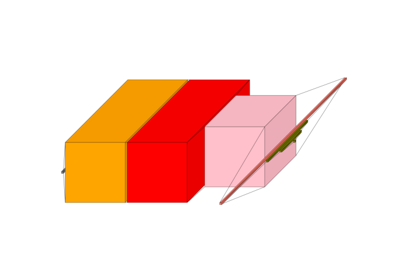
Visualkeras: Spam Classification Conv1D Dense Example
Visualkeras: Spam Classification Conv1D Dense Example